Emerging Opportunities with On-Device AI
The concept of on-device AI represents a shift from the traditional cloud-based approach that is likely to change the way we interact with our smartphones and tablets. Understanding the implications and transformative potential of local AI models can help companies offer improved mobile products to their users.
Introducing On-Device AI with Large Language Models (LLMs)
Traditionally, a large language model is hosted on powerful cloud servers, with mobile devices acting as clients, sending and receiving data over the internet. This approach, while functional, has inherent limitations:
- latency
- privacy concerns
- the need for a stable internet connection
In local language models, the LLM resides and operates directly on the user’s device, unlocking an array of new interesting possibilities. GenAI enables edge devices like smartphones, tablets, and PCs to process data locally, reducing the need for cloud data transmission and providing reliable offline capabilities.
Along with the rising popularity of artificial intelligence, companies like Apple, Google, or Qualcomm are developing their respective on-device machine learning capabilities and paving the way for AI integration on mobile devices.
Apple is pushing for more power with offline language models with OpenELM, Neural Engine, and Core ML frameworks. Google is also chasing after more powerful local language models with their Gemini Nano for on-device GenAI applications. Google's AI stack also includes AICore for mobile artificial intelligence integration with Android OS and Google AI Edge SDK, plus ML Kit (on-device machine learning). Qualcomm pursues local AI capabilities with AI Hub and their open-source AI models. These advancements can not not only enhance existing applications but also open the door for entirely new use cases and experiences.
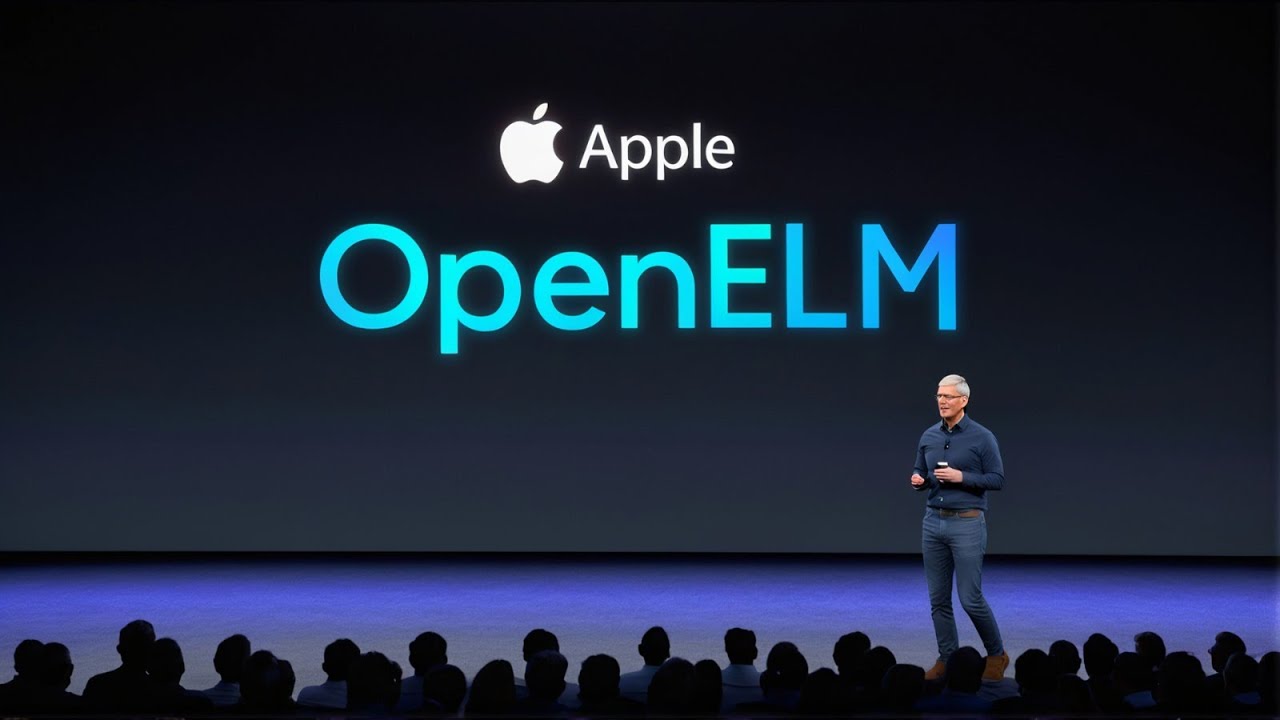
Advantages of On-Device AI for Edge Devices
The integration of LLMs onto mobile devices offers numerous advantages that will change the way we perceive and utilize AI in our daily lives.
Enhanced privacy and data security
By keeping sensitive data and AI processing on the device, users can enjoy greater privacy and security, as their personal information never leaves their mobile device. This is particularly crucial in industries like healthcare, finance, and government, where data privacy and compliance are of massive importance. The use of pre trained models for generative AI powered apps ensures that data processing is secure and efficient, directly on the user's device.
Offline functionality
With offline AI, users can access AI capabilities even in areas with limited or no internet connectivity, enabling seamless AI assistance in remote locations or during travel. This feature could be particularly valuable for industries like mining, exploration, and disaster response, where reliable connectivity is often a challenge.
Reduced Latency
Local processing eliminates the need to transmit data to remote servers, resulting in faster response times and a better user experience. The low-latency performance is critical for applications that depend on real-time responsiveness, such as augmented reality (AR), virtual reality (VR), Internet of Things (IoT), and gaming.
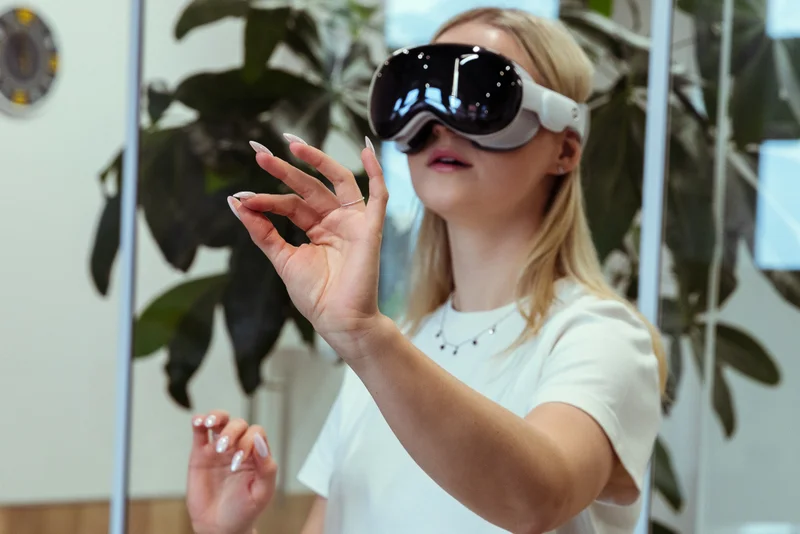
Cost Savings
Running AI on users’ devices can reduce cloud computing and data infrastructure costs for artificial intelligence companies, as they can leverage the idle computing power of customers’ devices. This cost-effective approach not only benefits businesses but also makes AI more accessible to smaller organizations and startups with limited resources.
Personalized Experiences
AI models perform better when they have access to personalized information about the user. A local AI model can leverage this data to provide more tailored and contextual experiences. By understanding the user’s preferences, habits, and contexts, these models can deliver highly relevant recommendations and assistance, ensuring accurate responses to user queries.
Developers can enhance user engagement by providing detailed instructions on leveraging models that run locally, ensuring users fully benefit from personalized and secure AI experiences.
Potential Risks and Challenges
While the benefits of on-device AI are undeniable, it is also crucial to address the potential risks and challenges associated with this technology.
Hardware Limitations
Current mobile devices may not have the computational resources or battery life to run resource-intensive LLMs efficiently, calling for further advancements in AI-specific hardware. However, it’s worth noting that companies like Qualcomm and Samsung are already working on developing dedicated AI chips and optimizing existing hardware for on-device AI workloads. The development of small models is crucial in this context, as they are designed to run efficiently on the limited hardware of mobile devices, addressing both computational and battery life concerns.

Model Optimization
Existing LLMs are often too large to run smoothly on mobile devices, requiring significant model compression and optimization techniques to ensure optimal performance. Researchers and developers are actively exploring techniques such as quantization, pruning, and knowledge distillation to reduce model size while preserving accuracy. The focus here is increasingly on optimizing local AI models that can operate directly on users' devices, enhancing data privacy and control by eliminating the need to send data back to the cloud.
Security Vulnerabilities
With AI models and sensitive data residing on the device, robust security measures will need to be implemented to prevent unauthorized access or data breaches. These could include, among other solutions, secure boot mechanisms, hardware-based encryption, and secure enclaves for isolating sensitive data.
Privacy Concerns
Although on-device AI promises enhanced privacy, users may still have concerns about the collection and use of their personal data, even if it remains on the device. To build trust and aid technology adoption, companies will need to introduce measures like transparent data practices, user control over data sharing, and robust privacy policies.
Fragmentation and Compatibility
Given the multitude of mobile devices, operating systems, and hardware configurations available on the market, it will be a challenge to ensure compatibility and consistent performance across a variety of platforms. This will call for device manufacturers, chipset vendors, and software developers to cooperate and come up with relevant industry standards.
Energy Efficiency
Running AI models on mobile devices can be energy-intensive and drain battery life quickly. Optimizing AI algorithms and hardware for energy efficiency will be a critical focus area to ensure a seamless user experience and widespread adoption of this new technology.
Despite these challenges, the potential benefits of on-device AI seem just too significant to ignore, and the industry is actively working to address these concerns through innovative solutions and collaborative efforts.
Mobile Apps That Will Benefit from On-Device AI
As on-device AI continues to gain traction, certain types of mobile applications are better positioned than others to reap the benefits of this emerging technology.
AR, VR, and IoT Apps
The high reliability and low latency of GenAI apps can enable new, valuable use cases for AR, VR and IoT applications in industries like healthcare, manufacturing, and logistics – that is, where seamless integration and real-time responsiveness are crucial. One such use case could include AR-powered smart glasses that can quickly recognize objects, provide contextual information, and guide a device-wearing technician in real-time through complex procedures and processes.
Healthcare Apps
On-device AI can improve users’ satisfaction with their healthcare apps by enabling real-time monitoring and analysis of vital signs, all while ensuring greater data privacy and usage of the user’s personal data already captured by and stored on the device. These intelligent apps could leverage on-device models to better detect anomalies, as well as providing bespoke recommendations for exercise and nutrition. In more advanced use cases AI could even assist medical professionals in making faster and more accurate diagnoses.
Productivity Apps
One area where on-device generative AI is sure to immediately prove valuable is the world of productivity. Switching from cloud-based to on-device AI operations could be an enabler for a plethora of new features, such as intelligent note-taking, automated message drafting, and context-sensitive meeting summarization. Imagine having a virtual assistant that can understand the context of your meetings, take notes, provide intelligent summaries, and even draft action items. All this while respecting your privacy and working offline when needed.
Financial Apps
Such apps can leverage on-device models to provide personalized financial advice, financial product recommendations, and intelligent virtual assistants tailored to each user’s unique circumstances, be they personal or business-related
Creative Tools
Artists, musicians, and content creators could leverage on-device AI for tasks like music generation, video editing, and creative writing.. With on-device AI, these creative tools can offer seamless integration with artists’ existing creative workflows that depend on other tools. Moreover, reduced latency will facilitate artistic expression that requires real-time, zero-delay cooperation between artists, also those working together remotely
Language Learning and Translation Apps
With powerful language models running on the device, language learning and translation apps can become more accurate and context-aware. This means providing real-time assistance and personalized feedback without the need for a stable internet connection, a feature bound to steal the hearts of all travelers.
Personal Assistants
With on-device AI, virtual assistants can become more intelligent, personalized, and context-sensitive. Relying on data stored on the user’s device could greatly enhance their ability to understand and assist users in various tasks and queries as they go about their day. This means, they could learn from the user’s preferences, habits, and contexts to provide truly tailored recommendations and assistance. Incorporating retrieval augmented generation can further enhance virtual assistants by enabling them to recover and re-rank documents or files in a chat interface, making them even more useful in managing daily tasks and information. All this while ensuring the highest degree of privacy protection and without the need for an uninterrupted internet connection.
Gaming and Entertainment
On-device AI could improve gaming experiences by enabling more intelligent and adaptive gameplay, realistic character interactions, and more personalized content recommendations. Similarly, entertainment apps could make the most of on-device AI by offering much better content curation that takes account of the user’s preferences, previous experiences, as well as other parameters, including age or even current mood.
Education Apps
With on-device AI, education apps can leverage local models to offer adaptive learning tailored to individual students’ needs, providing a personalized learning experience without the need for internet connectivity. They will also be able to rely on offline functionality for remote or low-connectivity areas and ensure that sensitive student data remains secure on the device.
Leveraging on-device AI to create more value for existing apps
As the adoption of on-device AI is picking up the pace, we can expect to witness a surge of innovative apps and novel use cases that will reshape the way we interact with our mobile devices. However, it’s worth emphasizing that the benefits of this exciting technology also apply to already-existing apps and the use cases that are now powered by cloud-based AI. This means companies can start building their AI solutions today and reap additional benefits tomorrow. The potential for scaling up on-device AI capabilities with larger models in the future opens up exciting possibilities for enhancing app performance and functionality. Currently, the use of small language models and even the small model option demonstrates the practical application of on-device AI, despite the fact that these smaller, more efficient models may run slowly on less powerful devices.
Fibo — nomtek's take on AI in education
Let’s now put aside theoretical scenarios and have a look at a real-world example. Here at nomtek we’re committed to life-long learning and the democratization of knowledge. To this end, our R&D department has recently developed Fibo – an education app that assists secondary and high-school students with understanding the fundamental concepts in mathematics (use case article pending). Along with chemistry and physics, math is considered to be one of the most challenging school subjects, one that it is also expensive to get quality private tutoring for.
Our team created a Flutter-based mobile app and enriched it with AI capabilities to provide free-of-charge tutoring at the level appropriate for the school-leaving exams held in several counties. Importantly, ChaptGPT was fine-tuned so that it actually assists in learning and understanding math concepts by way of offering explanations and providing feedback, rather than simply producing a Correct / Incorrect response to a set of exercises.
In this way, we built an AI math tutor app that could substitute for costly private lessons outside the classroom. These, arguably, have become a norm in a world where public education systems often leave teachers with insufficient time to fully explore important topics and to devote adequate attention to all students.
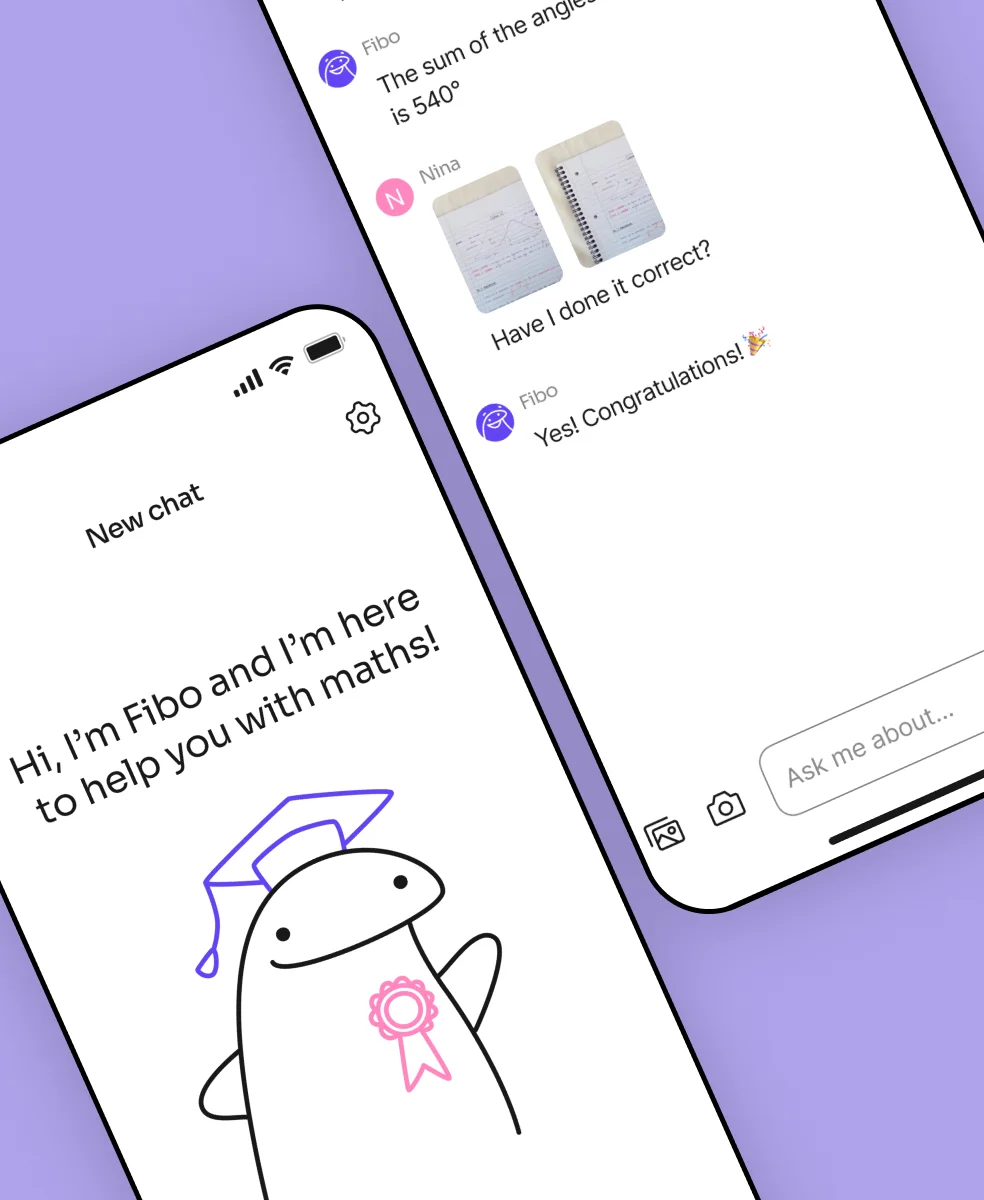
It’s easy to see that while we might have achieved our goal for students in certain geographies, those living in areas where internet access is limited and/or expensive are still left with no affordable options. This is precisely where on-device AI will prove immensely valuable, as students will be able to use tailored LLMs whenever and wherever they need, at no additional cost. In turn, for the development team, this means utilizing what has already been built and only putting some extra effort to reroute AI operations from cloud-based servers to the students’ devices.
By leveraging the power of AI models operating on students’ devices, Fibo – and a myriad of other apps – will be able to become as affordable and readily available as students’ personal tutors, thus supporting our and other companies’ mission to democratize learning.
Offline Machine Learning Models and Large language models Are the future of Mobile Experiences
On-device AI represents a paradigm shift in the world of mobile computing and AI. By bringing LLMs directly onto our smartphones and tablets, this technology promises to unlock new levels of privacy, efficiency, and personalized experiences. While challenges remain, the potential benefits are too significant to ignore. We are now in the early days of this technological transformation, but it is already clear that on-device AI will play a pivotal role in shaping the future of how we live, work, learn, and interact with the world around us.

Related articles
Supporting companies in becoming category leaders. We deliver full-cycle solutions for businesses of all sizes.